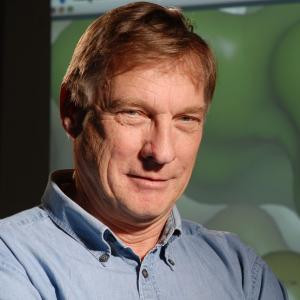
Contact
Email: jmoult@umd.edu
Call: (240) 314-6241
John Moult
Professor
Moult Group
Contact
Email: jmoult@umd.edu
Call: (240) 314-6241
Education
- D.Phil., Molecular Biophysics, University of Oxford, 1970
- B.Sc., Physics, University of London, 1965
Profile
Research in Dr. John Moult’s laboratory is focused on computational modeling of biological systems, including:
- Investigating the effects of missense single nucleotide variants on protein structure and function to elucidate their role in human disease
- Integrating knowledge of the biological mechanisms underlying the relationship between human genetic variation and disease, particularly complex trait diseases such as Alzheimer’s, diabetes, and Crohn’s disease
- Using novel neural network architectures derived from deep biological knowledge to probe aspects of disease mechanism, including the evaluation of potential drug targets and the best choice of drug for any patient, given their genome sequence
- Conducting large-scale community experiments to assess and advance the state of the art in areas of computational biology, particularly genome interpretation and protein structure modeling
CURRENT RESEARCH
Missense Single Nucleotide Variants and Disease
In more than 7000 rare Mendelian diseases (e.g. cystic fibrosis, sickle cell anemia) and in most cancers, the most common underlying genetic causes are changes of a single DNA base resulting in an amino acid substitution, in turn resulting in altered protein function. The Moult lab develops new computational methods to determine the impact of these variants, using machine learning together with evolutionary and protein structure information. Emphasis is on maximizing the number of cases in which interpretation meets the high standards of reliability required in the clinic.
Integrating Knowledge of Disease Mechanism
Sequencing of the human genome drove an explosion of research into the relationship between genetic variants and disease phenotypes. The resulting deluge of information is overwhelming and knowledge is scattered throughout the literature. Dr. Moult’s group has developed a formal description of biological mechanism and they are utilizing it to capture and represent what is known (and not known) for key genotype/disease phenotype relationships. The disease mechanism framework is being developed in collaboration with a philosopher of science, Professor Lindley Darden, Department of Philosophy, University of Maryland, College Park. Knowledge is entered and accessed through a web resource, www.MecCog.org.
Novel Neural Network Architectures for Studying Disease Mechanism
Integrated knowledge of mechanism provides a basis for new quantitative analyses of disease. To this end, the Moult lab is developing a new neural network protocol, in which the network architecture is dictated by the disease mechanism, and nodes represent system perturbations at different stages of biological organization (e.g. RNA, protein, cell, tissue). Networks are trained using data from disease-related, genome-wide association studies (GWAS). Unlike conventional neural networks, direct representation of mechanism allows many aspects of a disease to be probed, including evaluation of uncertain mechanism components, exploration of non-linear and emergent properties, evaluation of potential drug target efficacy, and how drug response will depend on the genetic variants present in a patient.
Community Experiments to Advance Computational Biology
Computational biology is an extremely rapidly moving field, with new large data sets and algorithms continually appearing. In parallel with this, the advent of facile electronic communications and database access facilitate a new form of science in which participants from around the world work on common problems. Community-wide critical assessment experiments, such as Critical Assessment of Structure Prediction (CASP) and Critical Assessment of Genome Interpretation (CAGI) provide a platform for assessing the state-of-the-art in each area rapidly and clearly, thereby driving progress.